PHD2024-14
Synthesizing Multimodal Data for Understanding Behavioral Transformations: A Deep Learning Approach
Host laboratory and collaborators
Olivier Manzoni, Inmed olivier.manzoni@inserm.fr
Stéphane Ayache, LIS stephane.ayache@lis-lab.fr
Rafika Boutalbi, LIS rafika.boutalbi@lis-lab.fr
Abstract
Our aim is to develop a robust quantitative heuristic tool using multimodal/multi-view deep learning approaches to identify behavioral transformations during lifetime. Our project leverages computational models to analyze and synthesize multimodal data sources, encompassing multi-view recordings of natural behavior (top and side views), 3D-imaging, 2D-tracking, ultrasonic vocalization, neuronal 3D structures, wireless EEG, and ex-vivo electrophysiology in both sexes in neurotypical and developmental models of psychiatric disorder (e.g., autism, perinatal stress). This project will unveil concealed patterns and relationships within these datasets, providing a comprehensive understanding of the interplay between genetics, behavior, and cellular physiology. The integration of longitudinal studies and sex difference with deep learning approaches is poised to expose hidden patterns and illuminate the dynamic nature of individual and group behaviors.
Keywords
Naturalistic behavior – Multiview clustering – Multimodal deep learning – Sex differences – Big data – Machine learning
Objectives
The main objectives are to 1/ develop unsupervised multi-view approach discovering behavior profiles according to sex and pathology 2/ uncover hidden relationships within datasets to understand sex-specific behavioral transformations over time and identify common patterns between behavior profiles 3/ develop a quantitative heuristic tool using multimodal deep learning to predict altered brain areas and/or neuronal circuits and 4/ to transfer methodologies to advance interdisciplinary research.
Proposed approach (experimental / theoretical / computational)
Experimental: We will generate and combine multiple day’s continuous multi-view video recording of freely interacting mice, datasets from combined 3D imaging and 2D-position tracking (i.e., Live Mouse Tracker), bioacoustic identification of ultrasonic communications (i.e., DeepSqueak), wireless EEG data and ex-vivo synaptic/cellular properties and biochemical markers. All data are collected according to a specific time stamp (see figure 1).
Computational: Based on multimodal (multi-view) data, we will develop novel unsupervised probabilistic multi-view approach to model the behavior of profiles of mouses with different pathologies. Based on data mining approaches we will explore common profiles characteristics. We will develop a multimodal deep learning approach and the discovered profiles predicting ex-vivo synaptic alterations in identifed brain areas based on behavior profiles.
Interdisciplinarity
This project is on the forefront of advancing (i) basic research by seeking fundamental knowledge in neurosciences and neurodevelopmental brain disorders, and (ii) utilizing modern multiview and multimodal deep learning methods. It distinctly surpasses the current state-of-the-art in these research domains. Leveraging its multidisciplinary nature and heuristic approach, the anticipated outcomes are poised to produce (i) a comprehensive set of experimentally derived data with an interdisciplinary character and (ii) a robust quantitative heuristic tool applicable to the broader neuroscience community. These findings are expected to significantly impact the understanding of fundamental brain functions, with potential implications for healthcare and the broader public domain.
Expected profile
- Robust theoretical background in computation, deep-learning and big data.
- Strong attraction for / background in neurosciences.
- Strong collaborative and positive team spirit.
- Good sense of humor.
Is this project the continuation of an existing project or an entirely new one? In the case of an existing project, please explain the links between the two projects
This is an entirely new project building up onto the previous discoveries of both supervisors’ laboratories.
2 to 5 references related to the project
- Z. Jiang et al., “Multi-View Mouse Social Behaviour Recognition With Deep Graphic Model,” IEEE Transactions on Image Processing, vol. 30, pp. 5490-5504, 2021, doi: 10.1109/TIP.2021.3083079.
- G. Giua, B. Strauss, O. Lassalle, P. Chavis, O. Manzoni, bioRxiv 2023.09.26.559495; doi: 10.1101/2023.09.26.559495.
- He, L., Li, H., Chen, M., Wang, J., Altaye, M., Dillman, J. R., & Parikh, N. A. (2021). Deep multimodal learning from MRI and clinical data for early prediction of neurodevelopmental deficits in very preterm infants. Frontiers in neuroscience, 15, 753033.
- Hügle, M., Kalweit, G., Hügle, T., & Boedecker, J. (2021). A dynamic deep neural network for multimodal clinical data analysis. Explainable AI in Healthcare and Medicine: Building a Culture of Transparency and Accountability, 79-92.
- Ye, J., Hai, J., Song, J., & Wang, Z. (2023). Multimodal data hybrid fusion and natural language processing for clinical prediction models. medRxiv, 2023-0
Two main publications from each PI over the last 5 years
- Badreddine Farah, Stéphane Ayache, Benoit Favre, Emmanuelle Salin. Are Vision-Language Transformers Learning Multimodal Representations? A Probing Perspective. AAAI 2022.
- Alice Delbosc, Magalie Ochs, Stéphane Ayache. Automatic facial expressions, gaze direction and head movements generation of a virtual agent. ICMI Companion 2022
- Iezzi D, Caceres-Rodriguez A, Chavis P, Manzoni OJJ. In utero exposure to cannabidiol disrupts select early-life behaviors in a sex-specific manner. Transl Psychiatry. 2022 Dec 5;12(1):501. Doi: 10.1038/s41398-022-02271-8.
- Bara A, Manduca A, Bernabeu A, Borsoi M, Serviado M, Lassalle O, Murphy M, Wager-Miller J, Mackie K, Pelissier-Alicot AL, Trezza V, Manzoni OJ. Sex-dependent effects of in utero cannabinoid exposure on cortical function. Elife. 2018 Sep 11;7:e36234. Doi: 10.7554/eLife.36234.
- Boutalbi, R., Labiod, L., & Nadif, M. (2021). Implicit consensus clustering from multiple graphs. Data Mining and Knowledge Discovery, 35, 2313-2340.
- Boutalbi, R., Labiod, L., & Nadif, M. (2020). Tensor latent block model for co-clustering. International Journal of Data Science and Analytics, 10(2), 161-175.
Project's illustrating image
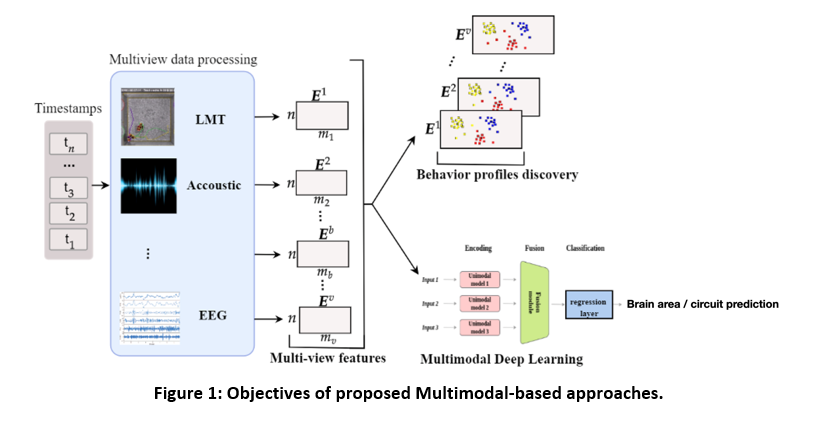