PHD2024-11
Modeling sensory neuron development
Host laboratory and collaborators
RUPPRECHT Jean-François, CPT jean-francois.RUPPRECHT@univ-amu.fr
LECUIT Thomas, IBDM thomas.lecuit@univ-amu.fr
Abstract
A distinctive feature of neurons is their elaborate tree-like structure (1). Such dendritic arbour morphology affects the type of inputs a neuron receives and the efficiency of their integration and is thus crucial for proper neural functioning (2). What sets the shape of these structures? For a long time, technical limitations have hampered the study of the dynamics of dendrite development in vivo. These limitations have been overcome recently in the Drosophila model system, revealing the growth of sensory neurons, which display complex dendritic arborizations. We study two classes of neurons (class I and IV) distinct by their size and complexity. This revealed that branches alternate stochastically between phases of growth, retraction and pausing following a class-specific probability distribution (3,4). In this project, we seek to test several hypotheses regarding how cytoskeletal regulators (e.g. actin and microtubules) and membrane tension underpin the stochastic switch between growth and shrinkage phases, as well as growth arrest. We will first finalize the extraction of the dynamical parameters from experimental imaging data. We will also develop a computational approach that encapsulates membrane tension together the diffusion/transport of regulators. In doing we will explore how the tree shape may feedback on these subcellular processes.
Keywords
Spatial statistics, Branching Annihilating Random Walks, Tissue morphogenesis, Pattern formation, Actin dynamics, Deep-Learning-based Image analysis, Reinforcement Learning,
Objectives
Specifically, this PhD proposal aims at
(1) extracting the microscopic parameters (e.g. branching rates) from experimental time-lapse images,
(2) correlating the variations in microscopic parameters with the location of specific molecular effectors,
(3) building models of branched networks that encapsulate cell mechanics, diffusion/transport of regulators
(4) predicting the effect of mechanical and biochemical perturbations based on these models
Proposed approach (experimental / theoretical / computational)
- Theoretical approach: Here we propose to develop a new Branching Annihilating Random Walk (BARW). Traditional BARW models, such as the one developed in (5), does not incorporate retraction phases, neither spontaneous nor contact-induced. In addition, the model was validated upon comparison to fixed image experimental data; the dynamics were inferred by comparing images from different embryos. We propose to consider a branching process whose properties are affected by the process's local density (à la McKean-Vlasov).
- Computational approach: We will use numerical simulations for the transport of molecular effectors and mechanical models (à la vertex model), with vertices regularly spaced along the neuronal structure. Several global statistics will be fitted to the experimental data set using a typical reinforcement learning approach.
Interdisciplinarity
This project is interdisciplinary at its heart and will involve analytical work (e.g. BARW), computational approaches (e.g. a modified vertex model), and computational aspects (e.g. deep-learning-based image analysis, with reinforcement learning tools for the parameter estimation).
Expected profile
We are looking for a passionate student who enjoys solving important puzzles. The applicant is expected to enjoy coding, simulations, dealing with experimental images, and performing analytical work. We target students in either interdisciplinary (e.g. quantitative Biology) or disciplinary (e.g. Physics, Computer Science, or Applied Maths) programs.
Is this project the continuation of an existing project or an entirely new one? In the case of an existing project, please explain the links between the two projects
This is a new proposal, but it builds on the groundwork laid by Marc-Eric Perrin. Marc-Eric developed the image analysis tools, which will allow for the inference of parameters and the subsequent development of more physical models.
2 to 5 references related to the project
- S. Ramón y Cajal, Histologie du système nerveux de l’homme et des vertébrés. (Maloine, Paris :, 1909)
- V. A. Kulkarni, B. L. Firestein, The dendritic tree and brain disorders. Mol. Cell. Neurosci. 50, 10–20 (2012).
- A. Palavalli, N. Tizón-Escamilla, J.-F. Rupprecht, T. Lecuit, Deterministic and stochastic rules of branching govern dendritic morphogenesis of sensory neurons, Current Biology (2020).
- S. Shree et al. Dynamic instability of dendrite tips generates the highly branched morphologies of sensory neurons, Science Advances (2023).
- E. Hannezo et al., A Unifying Theory of Branching Morphogenesis, Cell (2017)
Two main publications from each PI over the last 5 years
T. Lecuit:
- A Bailles, C Collinet, JM Philippe, PF Lenne, E Munro, T Lecuit, Genetic induction and mechanochemical propagation of a morphogenetic wave, Nature (2019).
- A. Palavalli, N. Tizón-Escamilla, J.-F. Rupprecht, T. Lecuit, Deterministic and stochastic rules of branching govern dendritic morphogenesis of sensory neurons, Current Biology (2020).
J.-F. Rupprecht:
- M. Prasad, N. Obana, S.-Z. Lin, K. Sakai, C. Blanch-Mercader, J. Prost, N. Nomura, J.-F. Rupprecht, J. Fattaccioli, A. S. Utada, Alcanivorax borkumensis Biofilms Enhance Oil Degradation By Interfacial Tubulation, Science (2023).
- A. Palavalli, N. Tizón-Escamilla, J.-F. Rupprecht, T. Lecuit, Deterministic and stochastic rules of branching govern dendritic morphogenesis of sensory neurons, Current Biology (2020).
Project's illustrating image
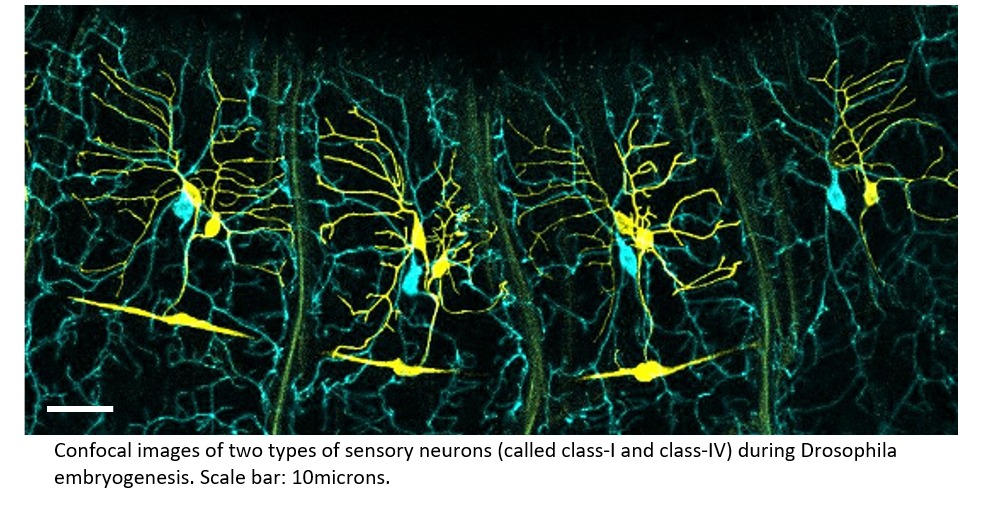